第六届中国数据挖掘会议(CCDM 2016)

- 参会报名
- 会议通知
- 会议论坛
- ACM中国年会
- 参会指南
第六届中国数据挖掘会议(CCDM 2016)已过期
|
发票类型:增值税普通发票 增值税专用发票 |
会议通知
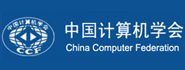
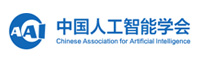
会议论坛
中国智能体及多智能体系研讨会
史忠植,中科院计算所,研究员安 波,南洋理工大学,副教授陈小平,中国科技大学,教授毛新军,国防科技大学,教授高 阳,南京大学,教授
讲者一: 史忠植 中科院计算所 ,研究员讲者简介:史忠植 中国科学院计算技术研究所研究员, 中国计算机学会会士,中国人工智能学会会士,IEEE高级会员,AAAI和ACM会员,IFIP人工智能学会机器学习和数据挖掘工作组主席。博士生指导教师。1968年毕业于中国科学院研究生院。长期从事智能科学、人工智能的研究。多次荣获中国科学院和国家科技进步奖。发表著作14部和学术论文450多篇。2013年获得中国人工智能学会吴文俊人工智能科学技术成就奖。担任《International Journal of Intelligence Science》主编。多次担任国际学术会议程序委员会主席或委员。曾担任中国人工智能学会副理事长、中国计算机学会秘书长。
讲者二: 安 波 南洋理工大学/中科院计算所讲者简介:安波是新加坡南洋理工大学计算机工程学院的南洋助理教授,于2011年在美国麻省大学Amherst分校获计算机科学博士学位。主要研究领域包括人工智能、多智能体系统、博弈论、及优化。有40余篇论文发表在人工智能领域的国际顶级会议AAMAS、IJCAI、AAAI、ICAPS以及著名学术期刊JAAMAS、IEEE Transactions。曾获2010年国际智能体及多智能体系统协会杰出博士论文奖,2011年美国海岸警卫队的卓越运营奖,2012年国际智能体及多智能体系统年会最佳应用论文奖,IAAI’2016创新应用论文奖,以及2012年美国运筹学和管理学研究协会(INFORMS)杰出运筹学应用奖等荣誉。他当选为国际智能体及多智能体系统协会理事会成员。演讲题目 :多智能体系统研究最新进展与热点研究方向摘要:多智能体系统(Multi-agent Systems)由分布式人工智能演变而来,其研究目的在于解决大规模、复杂、实时和有不确定信息的现实问题。经过近30年的发展,多智能体系统已经成为国际人工智能的前沿学科和研究热点。报告将讨论多智能体系统研究一些当前热点研究问题以及未来研究挑战。
5月20日上午8:30-9:20,史忠植报告5月20日上午9:20-10:10,唐平中报告TEA BREAK5月20日上午10:20-11:10,郝建业报告5月20日上午11:10-12:00,王崇骏报告LUNCH5月20日下午13:30-14:20,刘际明报告5月20日下午14:20-15:10,刘咏梅报告TEA BREAK5月20日下午15:20-16:10,安波报告5月20日下午16:10-17:00,秦涛报告5月20日下午17:10-18:00,博士生论坛5月20日下午18:00-18:30,学组工作会议
生物医学数据挖掘研讨会
讲者一: 吴信东 合肥工业大学,教授讲者简介:合肥工业大学教授、博士生导师,专业学者、海外杰青、IEEE Fellow、AAAS Fellow。演讲题目 :Pattern Matching and Mining with Wildcards and Length Constraints时长: 45分钟
讲者二: 周水庚 复旦大学,教授讲者简介:复旦大学计算机科学技术学院教授,上海市智能信息处理重点实验室副主任(2004-),曾任计算机科学与工程系副主任(2004/12-2008/4)。目前为中国计算机学会杰出会员、IEEE/ACM SIGMOD会员、中国计算机学会数据库专委会委员、大数据专委会等的委员。演讲题目 :基于数据挖掘的生物信息学研究时长: 45分钟摘要:数据挖掘是从大量数据中获取知识的主要技术手段。生物大数据的出现为数据挖掘提供了新的挑战与机遇。在这个报告中,我将介绍生物数据挖掘的特点与挑战性问题以及近年来我课题组运用数据挖掘方法开展的一些生物信息学研究课题与取得的结果。
讲者三: 张学工 清华大学,教授讲者简介:清华大学自动化系、医学院教授,合成与系统生物学中心副主任,国家杰出青年基金获得者,清华信息科学与技术国家实验室(筹)生物信息学部主任,生物信息学教育部重点实验室副主任。演讲题目 :生物组学数据挖掘中的模式识别问题初探时长: 45分钟
5月20日上午8:30-9:20,吴信东报告5月20日上午9:20-10:10,周水庚报告5月20日上午10:10-10:30,茶歇5月20日上午10:30-11:20,张学工报告
聚类分析研讨会
13:00-13:05论坛致辞于剑教授
13:05-14:05Applications of fuzzy clustering in regression models楊敏生教授Abstract-In this invited talk, there are three parts to be presented. In Part I, I talk about fuzzy cluster-wise regressions. In part II, step-wise possibilistic c-regressions are considered. In part III, I present the newly proposed change-point regression models using fuzzy clustering.Regression analysis is used in evaluating the functional relationship between the dependent and independent variables. Cluster-wise (or called switching) regression analysis is to embed clustering techniques into regression models. Since Zadeh (1965) proposed fuzzy sets that produced the idea of partial memberships described by membership functions, fuzzy set theory has been widely applied in clustering. For fuzzy clustering, the fuzzy c-means (FCM) algorithm, first proposed by Dunn (1974) and then extended by Bezdek (1981), is the most commonly used method. Since Quandt (1958 and 1960) and Chow (1960) initiated researches on switching regressions, it had been widely studied and applied. Hathaway and Bezdek (1993) first combined switching regressions with FCM by embedding fuzzy c-partitions to regression models, and referred to them as fuzzy c-regressions (FCRs). However, the FCRs are sensitive to noise and outliers. In part I, I present the work of Yang et al. (2008) that applied the concept of the -cut implemented clustering algorithms (FCM) to FCR and created the FCR algorithm to improve the robustness against noise and outliers, especially for c-regression models.Krishnapuram and Keller (1993) proposed a possibilistic c-means (PCM) clustering approach which is more robust than FCM to noise and outliers. Our current study is to embed possibilistic clustering into switching regression models and called it possibilistic c-regressions (PCR). Although PCR ameliorate the problem of outliers and noisy points more than FCR, PCR still depends heavily on initializations. In part II, I present the step-wise possibilistic c-regressions (SPCR) method which repeats PCR on a series of nested subsets using the clustering results of the previous subset as good initial values for PCR on the succeeding subset. The proposed SPCR is without initial values and robust to noises and outliers. Several experiments demonstrate that the accuracy and efficiency of our proposed method are superior to other c-regression methods.Change-point (CP) regression models have been widely applied in various fields where detecting change-points (CPs) is an important problem. Detecting the location of CPs in regression models could be equivalent to partitioning data points into clusters of similar individuals. In part III, I present a newly proposed method, called fuzzy CP (FCP) algorithm, for detecting the CPs and simultaneously estimate the parameters of regression models. The fuzzy c-partitions concept is first embedded into the CP regression models, and then it is transferred into the pseudo memberships of data points belonging to each individual cluster, and so these estimates for model parameters by the fuzzy c-regressions method can be obtained. Subsequently, the FCM clustering is used to obtain new iterates of the CPs collection memberships by minimizing an objective function concerning the deviations between the predicted response values and data values. Several numerical examples and real data sets are used, and experimental results show that the proposed FCP is an effective and useful CP detection algorithm for CP regression models.
14:05-15:05迁移原型聚类(Transfer Prototype-based Clustering)邓赵红教授Abstract—Traditional prototype-based clustering methods, such as the well-known fuzzy c-mean (FCM) algorithm, usually need sufficient data to find a good clustering partition. If available data are limited or scarce, most of them are no longer effective. While the data for the current clustering task may be scarce, there is usually some useful knowledge available in the related scenes/domains. In this study, the concept of transfer learning is applied to prototype-based fuzzy clustering (PFC). Specifically, the idea of leveraging knowledge from the source domain is exploited to develop a set of transfer prototype-based fuzzy clustering (TPFC) algorithms. First, two representative prototype-based fuzzy clustering algorithms, namely, FCM, and fuzzy subspace clustering (FSC), have been chosen to incorporate with knowledge leveraging mechanisms to develop the corresponding transfer clustering algorithms based on an assumption that there are the same number of clusters between the target domain (current scene) and the source domain (related scene). Furthermore, two extended versions are also proposed to implement the transfer learning for the situation that there are different numbers of clusters between two domains. The novel objective functions are proposed to integrate the knowledge from the source domain with the data in the target domain for the clustering in the target domain. The proposed algorithms have been validated on different synthetic and real-world datasets. Experimental results demonstrate their effectiveness in comparison with both the original prototype-based fuzzy clustering algorithms and the related clustering algorithms like multi-task clustering and co-clustering.
15:05-15:30茶歇
15:30-16:30Extended Variational Inference for Non-Gaussian Statistical Models马占宇副教授Abstract—Recent research demonstrate that the usage of non-Gaussian statistical models is advantageous in applications where the data are not Gaussian distributed. With conventionally applied model estimation methods, e.g., maximum likelihood estimation and Bayesian estimation, we cannot derive analytically tractable solution for non-Gaussian statistical models. In order to obtain closed-form solution, we extend the commonly used variational inference (VI) framework via lower-bound approximation, by utilizing convexity/relative convexity of the integrants in the non-Gaussian distributions. In this presentation, we introduce the principles of the extended variational inference (EVI) and demonstrate its advantages in non-Gaussian mixture models and bounded support matrix factorization. We also show the advantages of non-Gaussian statistical models in real life applications, such as speech coding, 3D depth map enhancement, and DNA methylation analysis. Here, we restrict our attention to the non-Gaussian distribution in the exponential family。
ACM中国年会
ACM数据挖掘中国年会-SIGKDD China Chapter Annual Conference
大会主席:杨强教授,香港科技大学周志华教授,南京大学
程序委员会主席:郑宇教授,微软亚洲研究院
活动具体流程(13:30-17:10):特邀报告(共五个,每个30分钟报告时间,5分钟提问)
报告人:杨强教授,香港科技大学计算机科学与工程系主任、KDD China主席摘要:大数据的本质是价值。人工智能为大数据价值的提取提供了很好的工具。 本讲座将通过实例,讲述如何通过大数据和数据挖掘来获得知识,从而更好地应用于不同的垂直领域。简介:杨强,ACM数据挖掘中国分会(KDD China)主席,香港科技大学计算机系主任,大学冠名讲座教授。他是国际人工智能协会(AAAI)的首个华人Fellow和IEEE等国际协会的Fellow,ACM杰出科学家。主要研究兴趣包括人工智能和数据挖掘,大数据,迁移学习与智能推荐。他指导的团队曾经赢得了ACM KDDCUP,诺基亚移动大赛等国际数据挖掘大赛的冠军。他是国际人工智能协会(IJCAI)的理事,中国人工智能协会常务理事,以及2015年国际人工智能大会(IJCAI 2015)的程序主席,系ACM Transactions on Intelligent Systems and Technology 和 IEEE Transactions on Big Data 的创刊主编,清华大学出版社出版的《学术研究,你的成功之路》一书的共同作者。于2012-2014年任华为诺亚方舟实验室创始主任, 现任微信-香港科技大学人工智能联合实验室主任等。
报告人:沈抖博士,百度公司网页搜索部高级总监、KDD China副主席摘要:搜索是数据挖掘相关技术应用最密集的互联网产品之一。从PC到移动,设别发生了巨大变化,用户使用搜索的行为也有很大迁移。如何应对这些变化并提供更好的搜索体验,是各个搜索引擎面临的巨大挑战。在这个报告中,我们会以最大的中文搜索引擎为例,探讨移动搜索的进展和机遇,并重点讨论数据挖掘技术在搜索中发挥的作用。简介:沈抖博士,ACM数据挖掘中国分会(KDD China)副主席,现任百度公司网页搜索部高级总监,入选“北京市海外高层次人才”,被聘为“北京市特聘专家”,在数据挖掘、信息检索、自然语言处理、人工智能、大数据等领域的多个国际顶级会议(SIGKDD、SIGIR、WWW、AAAI、IJCAI)和期刊(JMLR、TKDD、TOIS、TKDE、CACM)中担任主席、组委、评委、编委,先后发表了40余篇学术论文。
报告题目3:城市计算与大数据 (14:55-15:35)
报告人:郑宇教授 微软亚洲研究院主管研究员、KDD China秘书长摘要:城市计算是计算机科学以城市为背景,跟城市规划、交通、能源、环境、社会学和经济等学科融合的新兴领域。城市计算通过不断获取、整合和分析城市中不同领域的大数据来解决城市所面临的挑战。本报告将概述城市计算的定义、框架和主要研究问题,以典型应用为案例着重介绍大数据中跨域数据的融合和协同计算技术。具体案例包括基于大数据的细粒度空气质量分析和预测、城市油耗和汽车尾气排放评估,以及城市住房价值分级和评估等。相关技术发表在KDD等顶尖国际会议和期刊上,并在实际系统中部署应用。更多信息可参看城市计算主页:简介:郑宇(博士、教授、博导),ACM数据挖掘中国分会(KDD China)秘书长,微软亚洲研究院主管研究员、“城市计算”领域负责人,上海交通大学讲座教授、香港科技大学和香港理工大学客座教授;ACM Transactions on Intelligent Systems and Technology主编;担任过ICDE2014程序委员会主席和ICDM2015领域主席等10余次会议主席职务;在APEC2014 Smart City Forum、IE2014等10几个国际会议上做过大会主题报告;以主作者身份发表国际顶尖论文50余篇(近5年被引7900多次,2015年论文单年被引用2200余次,H-Index: 43);在ICDE2013和ACM SIGSPATIAL2010等知名国际会议上5次获得最佳论文奖;专著《Computing with Spatial Trajectories》被Springer评为(全球华人撰写的)最受欢迎的十本计算机类书籍之一;2013年全球杰出青年创新者(TR35)--《MIT科技评论评》;2013年现代创新者代表 --《时代》周刊;2014年中国40位40岁以下商界精英 -- 美国《财富》
报告人:漆远博士,蚂蚁金融服务集团的副总裁及首席数据科学家、KDD China委员摘要:蚂蚁金服的海量高价值数据为人工智能的技术发展和商业价值体现提供了坚实的基础。在这个报告中,我会介绍蚂蚁金服在基于大数据的人工智能方向上的一些最新进展,包括超大规模机器学习和深度学习,并讨论他们在客服,微贷和信用等业务的应用。简介:漆远博士为蚂蚁金融服务集团的副总裁及首席数据科学家。他的专业方向是大规模机器学习和贝叶斯推理。他是美国麻省理工媒体实验室博士,计算机与人工智能实验室博士后,普渡大学计算机科学系和统计系的终身教授。他曾访问MIT、剑桥大学、哥伦比亚大学、伦敦大学、杜克大学、SAMSI、布朗大学等多个高校和研究院的,受邀在各高校以及国际会议上发表众多演讲,并与IT、金融以及制药界研究者在机器学习以及数据分析问题上合作。 他是机器学习权威杂志Journal of Machine Learning Research的执行编辑以及机器学习会议ICML的领域主席,并获得微软牛顿研究奖和美国科学基金NSF Career奖。漆远博士是阿里巴巴与蚂蚁金服数据技术线负责人,目前致力于大规模机器学习和深度学习平台的建立及其在蚂蚁金服各项业务的应用。
报告人:蒋杰腾讯数据平台部总经理、KDD China委员摘要:通过比较几个主流要大数据机器学习平台,实现高效的分布式机器学习平台,需要构建一个能同时支持机器学习算法设计和大规模数据处理的一体化大数据机器学习系统。研究设计高效、可扩展且易于使用的大数据机器学习系统面临诸多技术挑战。简介:腾讯数据平台部总经理,腾讯T4大数据专家,863云计算课题腾讯主要负责人 ,CCF和广东省大数据专家委员会委员。拥有10年以上的大数据从业经验,之前在阿里巴巴工作5年,于2012年加入腾讯,负责腾讯分布式数据仓库、实时计算平台、精准推荐、信鸽、腾讯云分析等平台及产品的运营和研发工作,主要研究方向包括海量数据平台,人工智能和数据挖掘,分布式机器学习平台与智能推荐。
参会指南
【注】:a)全额代表、会员/学生代表注册费包含5月20-22日的中、晚餐(含一次宴会)、进入会场的权利、会议资料、合影留念等;陪同人员的注册费只包含5月20-22日的中、晚餐(含一次宴会);b)论文版面费由各期刊单独收取;c)会员代表是指:中国计算机学会会员、中国人工智能学会会员、ACM数据挖掘中国分会会员;学生代表是指:全日制在校本科生、硕士生和博士生,不含在职研究生和博士后;陪同人员是指陪同全额代表、会员代表或者学生代表参会的人员。
温馨提示酒店与住宿:为防止极端情况下活动延期或取消,建议“异地客户”与manbext客户端下载客服确认参会信息后,再安排出行与住宿。退款规则:活动各项资源需提前采购,购票后不支持退款,可以换人参加。
会员折扣
该会议支持会员折扣 具体折扣标准请参见plus会员页面会员返积分
每消费1元累积1个会员积分。 仅PC站支持。会员积分抵现
根据会员等级的不同,每抵用1元可使用的积分也不一样,具体可参见PLUS会员页面。 仅PC站支持。